Artificial Intelligence (AI) is revolutionizing industries worldwide, and at the heart of this transformation are four primary types of AI models: Generative AI, Predictive AI, Computer Vision AI, and Reinforcement Learning AI. Among these, Generative AI has taken center stage, powering breakthroughs in content creation, image synthesis, and automation. From ChatGPT and DALL·E to AI-powered video generation, Generative AI is reshaping how we interact with technology. But how does it compare to other AI models? In this guide, we break down the four types of AI models, how they work, and where they are most effectively applied.
You can also check out our simple explainer guide on what is artificial intelligence if you’re new to the topic.
Quick Overview of the 4 AI Models
To understand the broader AI landscape, let’s look at the four major AI models: Generative AI, Predictive AI, Computer Vision AI, and Reinforcement Learning AI.
AI Model | Primary Function | Common Applications |
---|---|---|
Generative AI | Creates new data based on patterns | AI-generated text, images, videos, and music |
Predictive AI | Forecasts outcomes based on historical data | Stock market predictions, fraud detection, healthcare analytics |
Computer Vision AI | Analyzes and interprets visual data | Facial recognition, self-driving cars, medical imaging |
Reinforcement Learning AI | Learns through trial and error to maximize rewards | Robotics, game AI, autonomous decision-making |
Generative AI?
Generative AI refers to artificial intelligence systems that create new content, such as text, images, audio, or even code. Unlike traditional AI models that analyze existing data and make predictions, Generative AI generates entirely new data based on learned patterns.
How Generative AI Works
Generative AI models, such as Large Language Models (LLMs) like GPT-4 and image generators like Stable Diffusion, rely on deep learning techniques, particularly neural networks. These models use training data to learn complex patterns and generate outputs that closely resemble human-created content.
At the core of Generative AI is deep learning, a subset of machine learning (check out our guide on Machine Learning vs Deep Learning to learn more about the differences) that relies on artificial neural networks to process vast amounts of data. These neural networks mimic the way the human brain processes information and recognize patterns.
Generative AI requires massive datasets to learn from. The model is fed with structured and unstructured data, including:
- Text-based AI Models (GPT-4, Claude, LLaMA) train on books, articles, conversations, and even code repositories.
- Image-based AI Models (Stable Diffusion, DALL·E, Midjourney) are trained on millions of images from the internet, learning how to replicate artistic styles, compositions, and textures.
- Music AI Models (AIVA, Jukebox) analyze musical compositions, enabling AI to generate original scores.
During training, the model learns to recognize patterns and develops probabilistic associations. For instance:
- In text models, AI predicts the next word based on statistical likelihoods.
- In image models, AI reconstructs pixels using diffusion processes.
Once trained, a Generative AI model can take user inputs (prompts) and generate responses through the following process:
- User Input: The AI receives a text prompt, image description, or keyword.
- Encoding: The input is transformed into a vector representation (numerical format for machine processing).
- Pattern Matching: The AI references its trained data to predict and generate the most likely next token, pixel, or musical note.
- Decoding: The output is refined iteratively, ensuring coherence, realism, and logical flow.
- For text generation, AI autocompletes sentences while maintaining style and factual consistency.
- For image generation, AI iteratively refines noise into a fully formed image (diffusion model process).
Real-World Applications of Generative AI
- Content Creation: AI-written blogs, books, and articles (e.g., ChatGPT, Jasper)
- Design & Art: AI-generated paintings, graphics, and photorealistic renders (e.g., Midjourney, Stable Diffusion)
- Programming: AI-assisted coding and debugging (e.g., GitHub Copilot)
- Entertainment: AI-generated music, deepfake videos, and film scripts
- Healthcare: AI-assisted drug discovery and medical imaging synthesis
The potential of Generative AI is vast, and as these models continue to evolve, they will become even more intelligent, context-aware, and capable of generating hyper-personalized content.
Advantages of Generative AI
- Creates high-quality, unique content quickly.
- Enhances automation and efficiency across industries.
- Enables personalization at scale (e.g., AI-generated marketing content).
Limitations of Generative AI
- Potential for misinformation and deepfake misuse.
- Requires vast computational power and large datasets.
- Can sometimes produce biased or inaccurate outputs.
Predictive AI
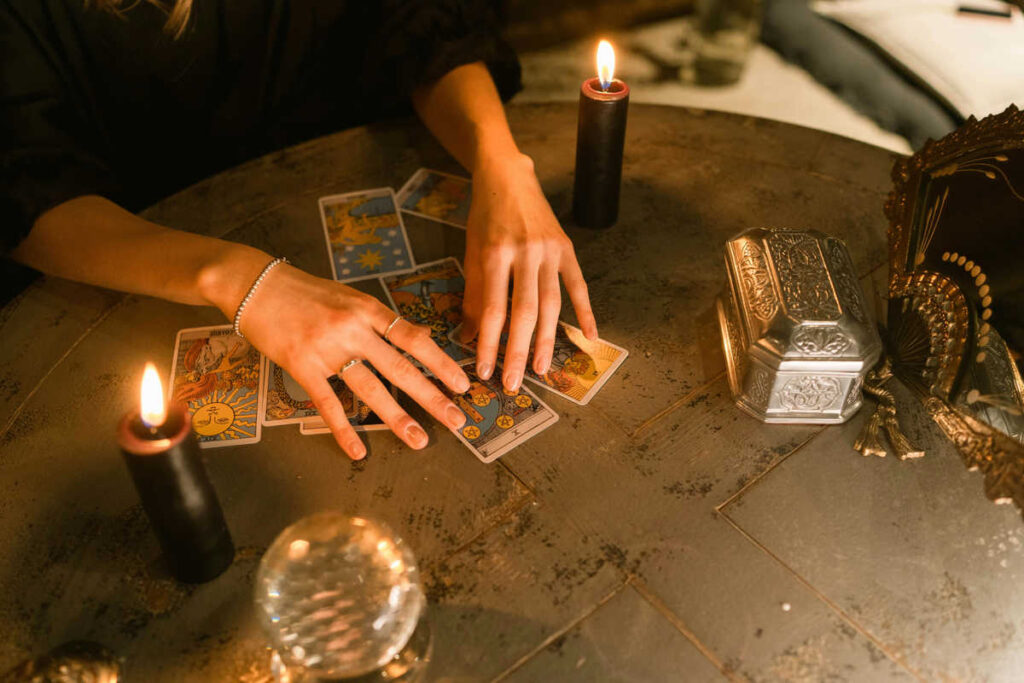
Predictive AI is one of the most widely used artificial intelligence models, designed to analyze historical data and forecast future outcomes with high accuracy. Unlike Generative AI, which creates new content, Predictive AI identifies patterns, makes probabilistic inferences, and optimizes decision-making across industries like finance, healthcare, retail, and cybersecurity.
How Predictive AI Works
Predictive AI relies on machine learning (ML) algorithms and statistical models to process historical data and identify trends. These models analyze past behaviors, detect patterns, and make predictions based on probabilistic reasoning.
The key techniques that power Predictive AI include:
Regression Analysis
Determines relationships between variables and predicts numerical outcomes (e.g., stock prices, sales revenue).
📌 Example: Predicting the price of Bitcoin based on market trends.
Classification Models
Categorizes data into predefined groups (e.g., fraudulent vs. non-fraudulent transactions).
📌 Example: Spam email detection in Gmail.
Time-Series Forecasting
Uses historical time-based data to predict future trends (e.g., weather forecasting, sales projections).
📌 Example: Netflix predicting which shows will trend based on past viewership patterns.
Neural Networks for Pattern Recognition
Deep learning models mimic human brain activity to improve accuracy in identifying non-linear relationships.
📌 Example: AI detecting early signs of disease from medical records.
Common Applications
Predictive AI is widely deployed across industries, driving efficiency and data-driven decision-making.
Finance & Banking
- Stock Market Predictions: AI-driven algorithms forecast stock trends based on historical market movements.
- Fraud Detection: Banks use Predictive AI to flag suspicious transactions in real time.
📌 Example: JPMorgan Chase uses AI models to detect credit card fraud by analyzing spending patterns and blocking unauthorized transactions instantly.
Healthcare & Medicine
- Disease Prediction: AI models assess patient history to predict disease risks.
- Medical Diagnosis: AI improves early detection of cancer, heart disease, and Alzheimer’s.
📌 Example: IBM Watson Health predicts the likelihood of cancer recurrence in patients, allowing for early intervention.
E-commerce & Retail
- Customer Behavior Forecasting: AI predicts purchasing trends, helping businesses optimize inventory.
- Personalized Recommendations: AI tailors product suggestions based on browsing history.
📌 Example: Amazon’s recommendation engine predicts what customers are likely to buy next based on past purchases.
Cybersecurity & Fraud Prevention
- Threat Detection: AI identifies anomalies in network traffic, preventing cyberattacks.
- Identity Verification: AI predicts fraudulent login attempts based on user behavior.
📌 Example: PayPal uses Predictive AI to prevent unauthorized transactions by analyzing geolocation, device fingerprinting, and transaction history.
Computer Vision AI
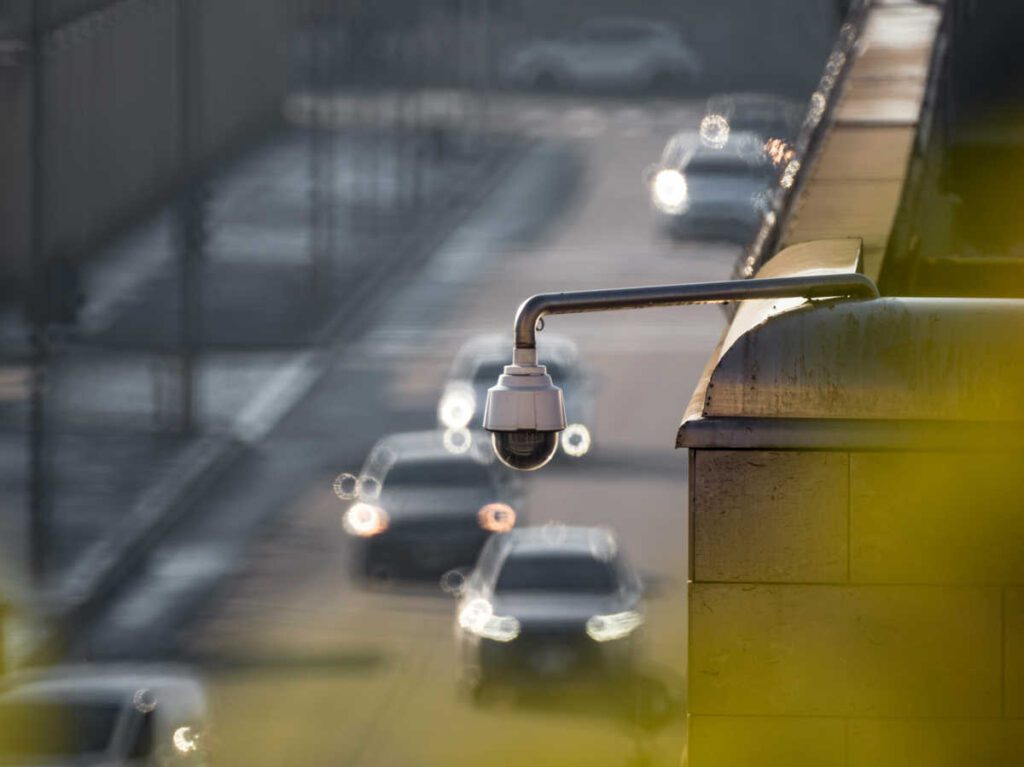
Computer Vision AI is an advanced field of artificial intelligence that enables machines to see, interpret, and process visual information similarly to humans. By leveraging deep learning and neural networks, Computer Vision AI powers everything from facial recognition and self-driving cars to medical imaging and industrial automation.
Unlike Generative AI, which creates new content, and Predictive AI, which forecasts outcomes, Computer Vision AI extracts meaningful insights from images and videos, enabling machines to make real-time, visual-based decisions.
How Computer Vision Works
At its core, Computer Vision AI is built on the interpretation of pixel-based data. It analyzes images and video frames, detecting patterns, objects, and even emotions within visual content.
Convolutional Neural Networks (CNNs)
CNNs are specialized deep learning architectures designed for processing visual data.
They break down images into layers of pixels and detect edges, textures, colors, and objects.
📌 Example: Facebook’s facial recognition system uses CNNs to identify people in photos.
Object Detection Algorithms
Models like YOLO (You Only Look Once) and Faster R-CNN scan images in real-time to detect multiple objects.
📌 Example: Self-driving cars use object detection to recognize pedestrians, traffic lights, and road signs.
Image Segmentation
Instead of identifying an object as a whole, segmentation AI divides an image into meaningful sections (e.g., separating a tumor from healthy tissue in a medical scan).
📌 Example: AI-powered diagnostic tools analyze MRI scans to detect diseases.
Optical Character Recognition (OCR)
OCR allows AI to extract text from images, turning scanned documents into editable digital text.
📌 Example: Google Lens translates text from signs or documents in real time.
Common Applications
Computer Vision AI is transforming industries by making machines capable of visual perception and automated decision-making.
Healthcare & Medical Imaging
- AI-powered Disease Detection: Computer Vision AI scans X-rays and MRIs to identify diseases like cancer, pneumonia, and diabetic retinopathy.
- Surgical Assistance: AI enhances robotic-assisted surgeries by providing real-time imaging.
📌 Example: Google’s DeepMind developed an AI system that diagnoses eye diseases with greater accuracy than human doctors.
Self-Driving Cars & Transportation
- Lane Detection: AI identifies road markings to keep vehicles within lanes.
- Obstacle Avoidance: AI-powered object detection helps prevent accidents.
📌 Example: Tesla’s Autopilot system uses Computer Vision AI to analyze traffic and navigate roads autonomously.
Retail & E-commerce
- Smart Checkout Systems: AI-powered stores allow customers to grab items and leave without checking out (e.g., Amazon Go).
- Virtual Try-Ons: AI enables customers to preview clothing, makeup, or glasses digitally.
📌 Example: Sephora’s AI lets customers test makeup products virtually before purchasing.
Security & Surveillance
- Facial Recognition: AI matches facial features to identify individuals.
- Anomaly Detection: AI detects unusual behavior in crowded places.
📌 Example: Airports use AI-powered facial recognition to enhance security screenings.
Reinforcement Learning AI
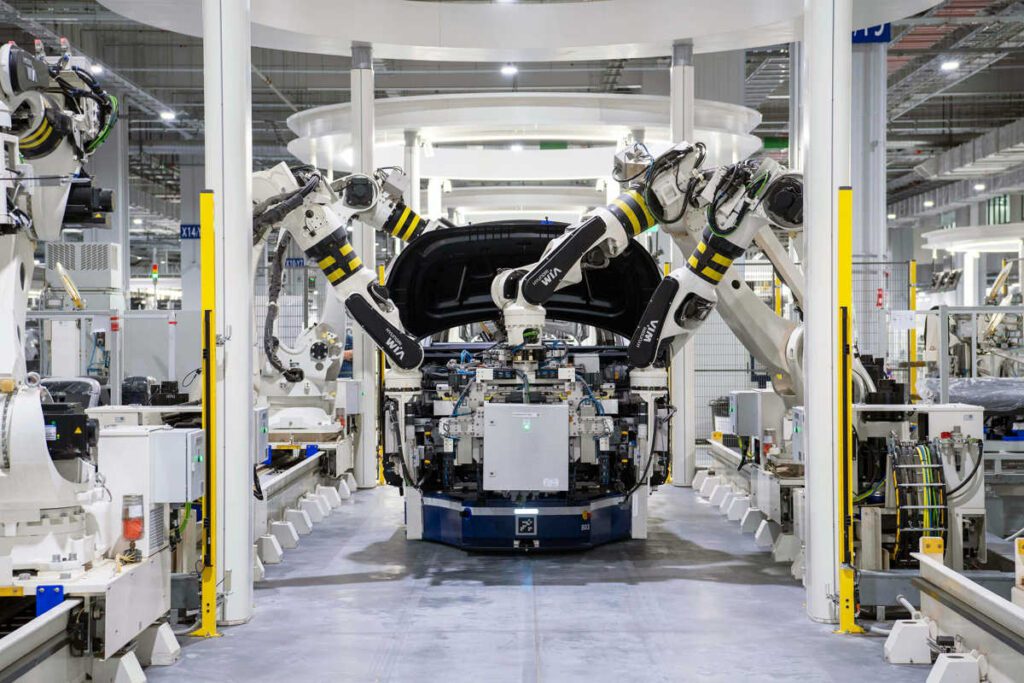
Reinforcement Learning AI (RL) is a specialized branch of artificial intelligence where models learn through trial and error, optimizing decisions based on rewards and penalties. Unlike Generative AI, which creates new content, or Predictive AI, which forecasts future outcomes, Reinforcement Learning AI focuses on decision-making in dynamic environments—making it the backbone of robotics, autonomous systems, gaming, and financial trading.
RL models don’t just process historical data like Predictive AI; they interact with environments, make choices, and improve over time based on feedback. This self-learning approach allows AI to perform tasks that require long-term planning, adaptability, and optimization.
Reinforcement Learning AI is inspired by behavioral psychology—just like humans learn through experience, rewards, and consequences, AI models improve their decision-making through reinforced feedback loops.
- Agent – The AI model that learns and makes decisions (e.g., a self-driving car or a game-playing bot).
- Environment – The system in which the agent operates (e.g., a chessboard, a stock market, or a robotic workspace).
- Actions – The choices the agent can make (e.g., moving left or right in a video game).
- Rewards & Penalties – Positive or negative reinforcement based on the agent’s decisions (e.g., winning points for correct moves, losing points for mistakes).
- Policy – The strategy the AI develops over time to maximize rewards and minimize penalties.
📌 Example: An AI-powered robot learns to walk by adjusting its balance. Every time it falls, it is penalized, but when it stays upright, it gets rewarded. Over time, the robot learns the optimal way to walk.
How Reinforcement Learning Works
Step-by-Step Process of Reinforcement Learning AI:
- Observation & State Recognition – The AI agent perceives its environment and its current state.
- Decision Making (Action Selection) – The AI takes an action based on its current knowledge (policy).
- Reward or Penalty – The AI receives feedback (positive or negative) based on its action.
- Policy Update (Learning & Optimization) – The AI refines its strategy to improve future decisions.
- Iteration & Continuous Learning – The AI repeats this cycle thousands or millions of times, refining its approach.
📌 Example: An AI-powered chess bot plays against itself millions of times, refining its strategy until it reaches grandmaster-level gameplay.
Common Applications
Reinforcement Learning AI is reshaping industries by enabling autonomous decision-making and real-time adaptability.
Robotics & Automation
- Autonomous Robots: AI-driven robots optimize their movements for industrial automation.
- Self-Learning Drones: AI adapts to flight conditions for delivery and surveillance tasks.
📌 Example: Boston Dynamics’ robots use RL to balance, walk, and navigate rough terrain.
Gaming & Simulation AI
- AI-powered NPCs (Non-Playable Characters) that adapt to player behavior.
- Game AI that learns from players to offer better challenges.
📌 Example: OpenAI’s RL-based AI defeated human eSports champions in Dota 2 by learning advanced strategies.
Self-Driving Cars & Transportation
- Traffic Signal Optimization: AI learns to minimize congestion by adjusting traffic light timing.
- Autonomous Vehicles: AI adapts driving behavior based on road conditions.
📌 Example: Tesla’s self-driving AI improves through reinforcement learning, continuously adjusting based on real-world driving feedback.
Finance & Algorithmic Trading
- Stock Market AI: RL-based trading bots predict market fluctuations and optimize investment strategies.
- Portfolio Optimization: AI dynamically adjusts asset allocations to maximize returns.
📌 Example: Hedge funds use RL-based AI models to make high-frequency trading decisions in milliseconds.
Healthcare & Drug Discovery
- Medical Treatment Optimization: AI recommends the best treatment plans for patients.
- Drug Discovery: AI tests new pharmaceutical compounds to find promising drugs faster.
📌 Example: AI-driven RL models accelerate cancer treatment research by optimizing drug interactions.
Which AI Model is Best for Your Use Case?
Choosing the right AI model depends on your specific needs. Here’s a quick guide:
- Choose Generative AI if… You need content creation, AI-generated images, or chatbot interactions.
- Choose Predictive AI if… You need forecasting, data-driven decisions, or fraud detection.
- Choose Computer Vision AI if… You need image recognition, security, or medical imaging solutions.
- Choose Reinforcement Learning AI if… You need autonomous decision-making, robotics, or AI-driven gaming.
Future of AI Models & Emerging Trends
As artificial intelligence continues to evolve, AI models—Generative AI, Predictive AI, Computer Vision AI, and Reinforcement Learning AI—are becoming more sophisticated, adaptive, and integrated. The future of AI will see these models working together, creating multi-modal AI systems that can think, learn, and create in ways that mimic human intelligence.
Here’s a look at the emerging trends and advancements shaping the future of AI models.
Generative AI’s Expanding Role
Generative AI is already revolutionizing content creation, but its next phase will push beyond text and image generation into multi-modal AI, advanced creativity, and real-time personalization.
What’s Next for Generative AI?
✅ Multi-Modal Generative AI – AI models will seamlessly integrate text, images, audio, and video into a single system. Future AI will generate interactive 3D environments, hyper-realistic AI avatars, and dynamic virtual worlds.
📌 Example: Imagine an AI that can take a text prompt and generate a fully interactive, game-like world in real time.
✅ AI-Powered Creativity & Co-Creation – Generative AI will move beyond passive content creation to collaborate with humans, offering real-time suggestions for writing, art, and music.
📌 Example: AI tools will help musicians compose songs by generating instrumental tracks based on a few melody inputs.
✅ Personalized AI-Generated Content – Future AI models will be capable of real-time content adaptation, creating custom AI-generated videos, articles, and interactive experiences tailored to individual users.
📌 Example: AI-driven news platforms that generate personalized reports based on user preferences, writing style, and even tone.
✅ AI-Generated Virtual Humans – AI models like Synthesia and DeepBrain AI are already creating hyper-realistic digital humans. The future will see AI-driven avatars that can hold real-time conversations and express emotions.
📌 Example: AI-powered virtual influencers will interact with audiences in real-time, creating fully digital brand ambassadors.
The Evolution of Predictive AI: Real-Time Decision Intelligence
Predictive AI will become faster, smarter, and more autonomous, making real-time, self-learning predictions that continuously refine themselves without human intervention.
What’s Next for Predictive AI?
✅ Real-Time Adaptive AI Models – Predictive AI will no longer rely solely on historical data but will make instant adjustments based on live data streams.
📌 Example: AI in stock trading will analyze global market conditions in real time and adjust investment strategies on the fly.
✅ AI-Powered Autonomous Business Forecasting – Companies will use AI to automate market analysis, supply chain management, and financial forecasting, allowing businesses to react instantly to economic shifts.
📌 Example: AI will predict consumer demand and adjust supply chains dynamically to prevent shortages or overstocking.
✅ Explainable AI (XAI) & Ethical AI – Predictive AI models will become more transparent and interpretable, ensuring businesses and governments can trust AI-driven decisions.
📌 Example: Future AI credit-scoring models will provide explanations for why a loan was approved or denied, reducing AI bias.
✅ AI-Powered Healthcare Predictions – AI will predict disease outbreaks, patient deterioration, and personalized treatment plans with near-perfect accuracy.
📌 Example: AI will analyze genetic data and lifestyle habits to predict an individual’s risk for diseases and offer preventive recommendations.
The Next Leap in Computer Vision AI: Machines That “See & Understand” Like Humans
Computer Vision AI is evolving from basic image recognition to full-scale video intelligence, spatial awareness, and self-adaptive vision models.
What’s Next for Computer Vision AI?
✅ AI-Powered Augmented Reality (AR) & Mixed Reality (MR) – Future AI-driven AR will seamlessly blend digital and physical environments, enhancing real-world interactions.
📌 Example: AI-powered smart glasses will recognize objects, translate text, and provide contextual information in real time.
✅ Self-Supervised Learning in Vision AI – Instead of relying on manually labeled datasets, AI will learn to recognize objects on its own, significantly reducing training time and improving accuracy.
📌 Example: AI-powered cameras will automatically detect suspicious behavior in real-time security surveillance.
✅ AI-Powered Smart Cities – Computer Vision AI will help monitor traffic, detect infrastructure issues, and enhance urban planning.
📌 Example: AI traffic cameras will dynamically adjust traffic light timings to prevent congestion.
✅ Medical Imaging AI Beyond Diagnosis – AI will assist in real-time robotic surgeries, early disease detection, and personalized medicine.
📌 Example: AI-powered microscopes will detect cancer cells more accurately than human pathologists.
The Future of Reinforcement Learning AI: Autonomous, Self-Learning AI Agents
Reinforcement Learning AI is already behind self-driving cars, robotics, and game AI, but the future will see RL-driven autonomous decision-making systems in every industry.
What’s Next for Reinforcement Learning AI?
✅ Self-Adaptive AI Agents – Future AI agents will learn from experience in real time, constantly refining their behavior without pre-programmed rules.
📌 Example: AI customer service agents will learn from interactions and improve their responses over time without human intervention.
✅ Reinforcement Learning in Robotics – Robots will learn complex, human-like tasks through trial and error, improving their adaptability.
📌 Example: AI-powered warehouse robots will autonomously reorganize inventory based on demand forecasts.
✅ Autonomous AI-Powered Factories – RL will drive the next industrial revolution, optimizing manufacturing without human supervision.
📌 Example: AI-driven factories will adjust production lines in real time to reduce waste and maximize efficiency.
✅ AI-Powered Game & Simulation Agents – Reinforcement Learning will power AI-driven NPCs (non-playable characters) that learn and adapt to human players, making games more immersive.
📌 Example: Future video games will feature AI-powered enemies that evolve based on player behavior, creating infinite replayability.
The Rise of Multi-Modal AI: When AI Models Work Together
The biggest trend in AI’s future is multi-modal AI, where different AI models—Generative AI, Predictive AI, Computer Vision AI, and Reinforcement Learning AI—are combined into one unified intelligence.
What’s Next for Multi-Modal AI?
✅ AI That Listens, Sees & Creates Simultaneously – AI will process voice, vision, and text simultaneously, enabling true conversational AI that understands real-world context.
📌 Example: AI-powered personal assistants will see what’s on your screen, listen to your commands, and generate responses accordingly.
✅ Generative AI + Computer Vision AI = AI-Generated 3D Worlds
📌 Example: AI will scan real-world environments and instantly create realistic 3D virtual spaces for gaming, simulations, and metaverse applications.
✅ Predictive AI + Reinforcement Learning AI = Self-Learning Financial Systems
📌 Example: AI trading bots will not only predict stock trends but also adapt their strategies dynamically based on global market shifts.
✅ AI-Powered Digital Twins – AI models will create real-time simulations of physical objects, buildings, and even human behavior, enabling predictive analysis at an unprecedented scale.
📌 Example: AI-powered smart cities will simulate traffic patterns to optimize urban planning in real time.
Final Thoughts
AI continues to evolve, and Generative AI leads the charge in revolutionizing content creation and automation. However, it works alongside Predictive AI, Computer Vision AI, and Reinforcement Learning AI to create a robust AI ecosystem. Understanding these four AI models will help businesses and individuals harness the power of AI effectively.
As AI technology advances, we can expect even deeper integration across these models, shaping the future of automation, creativity, and problem-solving.
Frequently Asked Questions (FAQ)
What is Generative AI, and how does it work?
Generative AI is a type of artificial intelligence that creates new content, such as text, images, or music, by learning patterns from existing data.
How is Generative AI different from Predictive AI?
Generative AI creates new data, while Predictive AI forecasts future trends based on historical data.
What are the 4 main types of AI models?
The four main types of AI models are Generative AI, Predictive AI, Computer Vision AI, and Reinforcement Learning AI.
Which AI model is best for my business?
It depends on your needs. Generative AI is best for content creation, Predictive AI for forecasting, Computer Vision AI for image analysis, and Reinforcement Learning AI for autonomous systems.